BACK TO RESEARCH WITH IMPACT: FNR HIGHLIGHTS
BACK TO RESEARCH WITH IMPACT: FNR HIGHLIGHTS
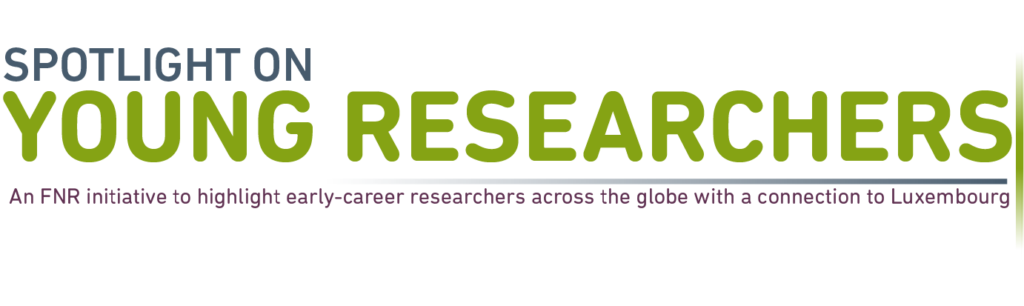
While machine learning and deep learning have come a long way, they are not yet at a stage where autonomous vehicles can handle unexpected situations. As part of a public research-industry collaboration, early career researcher Steve Dias Da Cruz investigates possibilities to reduce the amount of data needed to train reliable deep learning models for safety critical applications in the automotive industry.
Deep learning-based approaches are still like black boxes – it is difficult to provide interpretations and to give guarantees for safety-critical applications.
“Regarding autonomous vehicles, machine learning-based approaches have caused several fatalities in the last few years, because the systems did not behave as expected in some challenging situations. We will need to improve our understanding of such systems and to develop the necessary mathematical proofs to increase the safety, even – and particularly – when unforeseeable situations occur,” explains researcher Steve Dias Da Cruz, adding:
“I hope that some of my investigations will make a tiny step towards improvements of our understanding of these systems in order to bring machine learning models to practical applications where they can improve our daily lives safely.”
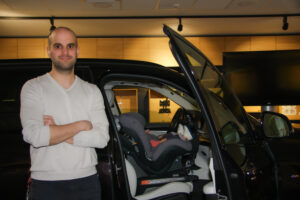
Infallible algorithms prerequisite for safe autonomous cars
“If machine learning, and in particular deep learning-based algorithms are to be used for safety critical applications in the automotive industry – for example during the airbag deployment in case of an accident – then it is important to ensure that you can trust the models you are using.”
Steve explains that the issue is that these approaches need vast amounts of data to work well. This means it is expensive and time consuming for a company to record the necessary data to develop a system for each new potential application. For the automotive industry, this would require a data collection process to be repeated for each new car model and automotive manufacturer – and this for each product. This would need to be done to ensure car models behave correctly in all possible scenarios.
“In my PhD project, I am trying to find ways to reduce the amount of data needed for training reliable deep learning models. On the one hand side, we want to evaluate the integration of simulated data. The benefit of the latter is the possibility to have full control about the entire data generation process, to get the annotations for free (which is sometimes more difficult than collecting the data in the first place) and to generate more data with a higher variability, which sometimes would not be possible with real data.
“On the other hand, we will investigate new training approaches to improve the reliability of machine learning models by dedicated design choices and we will try to corroborate these results with mathematical derivations.”
One public research, two industry partners
Steve’s PhD project is a public research-industry collaboration involving the University of Kaiserslautern, IEE S.A. and the German Research Center for Artificial Intelligence (DKFI), funded through the FNR’s Industrial Fellowship scheme.
Steve spends two out of every three weeks at IEE in Luxembourg, where he is based with a small research team working on different basic research topics. Every third week he is at the DFKI in Kaiserslautern, where he is part of a large group of PhD students working on state-of-the-art machine learning applications in computer vision.
“In this framework, I am able to learn and discuss with colleagues from two different departments and profit from the benefits of both worlds. I learn a lot from this interdisciplinary environment and there is always someone who knows the answers to the questions you might encounter during you research. I can share my experience with the colleagues on both teams and provide insights from industry to academia and vice versa, such that interesting collaborations can be developed.”
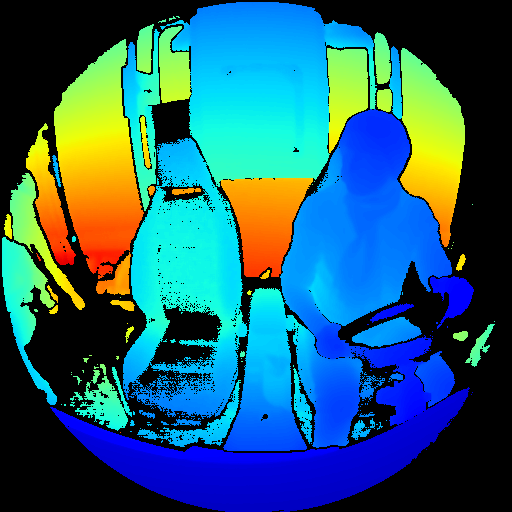
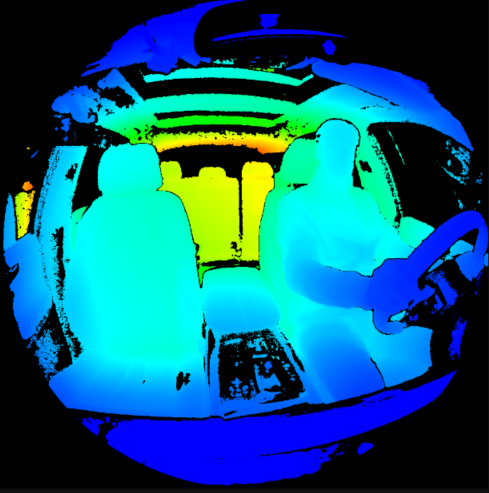
“Especially when you come from a more theoretical background as me, you learn about engineering constraints you did not know existed”
Being embedded in an industrial setting is a different experience from pure academia. In line with the majority of researchers who have experienced academia and industry, Steve explains the main difference is that in industry you are working on concrete solutions for a business.
“In industry, you learn about the expectations of customers and requirements by international regulations which need to be fulfilled by industry. These expectations need to be included as constraints during the development of possible solutions, which will make you think about problems from a new perspective. For example, you need to make sure that you fulfil safety regulations while deploying your algorithms for small devices with less computational power than your computer.
“Moreover, you get the chance to work on real data and on products which are in development for future deployment in millions of vehicles to improve the safety of the passengers.”
“Working together closely with the industry enables me to get hands-on experience with the problems the industry is facing – and I can learn from experienced scientists who have been doing applied research for many years. Especially when you come from a more theoretical background as me, you learn about engineering constraints you did not know existed. Since it was clear for me that I wanted to work on applied research afterwards, it is a valuable experience which I could not have obtained in a purely academic environment.”
It is not only the researchers who benefit from applying their expertise in industry – the company gets access to specific research skills they may otherwise not have.
“I think that the industry benefits form researchers like me in the sense that we can focus on investigations and basic research questions which are not involved with day-to-day business topics.
“We can investigate promising new technologies, spend more time on exploring different approaches and bring (hopefully) new perspectives to the group. On the other side, the close contact with academia enables both parties to learn from each other and to detect possible future collaborations.”
A synthetic dataset for occupancy detection
Despite being early in his PhD, Steve has already helped create a synthetic dataset for occupancy detection and classification in the vehicle interior. The team made the dataset publicly available so that investigations during his PhD project can be reproduced by the research community. Moreover, the team combines it with a public benchmark so that other researchers can evaluate their methods when machine learning models are trained under challenging, but realistic, conditions.
“Since it is publicly available, researchers can compare their results against other researchers’ approaches. The problem statement of our dataset is close to a real application and we hope to raise the awareness about some of the problems industry is facing regarding the deployment of the potential of machine learning models into practice.”
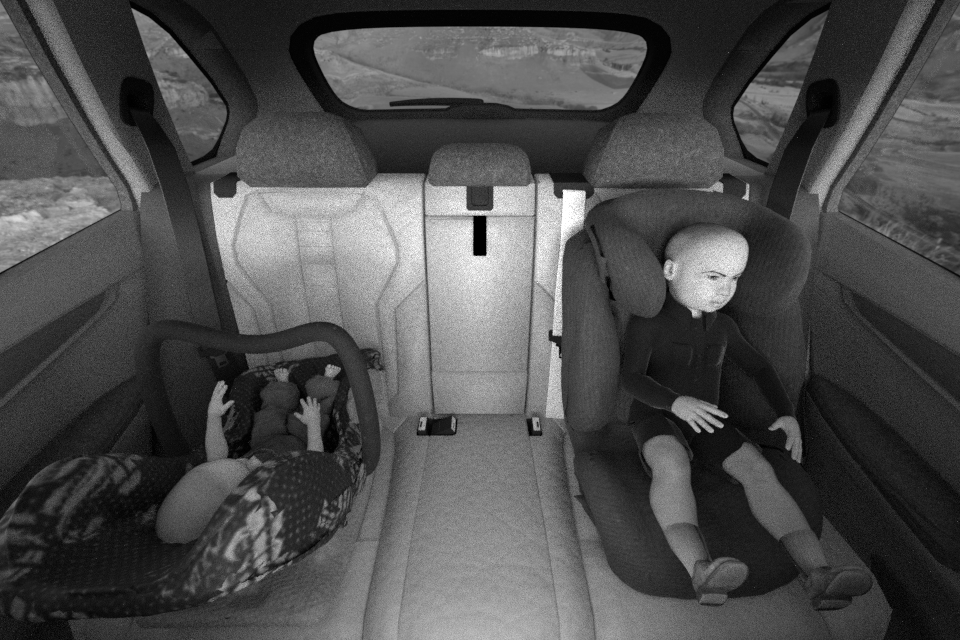
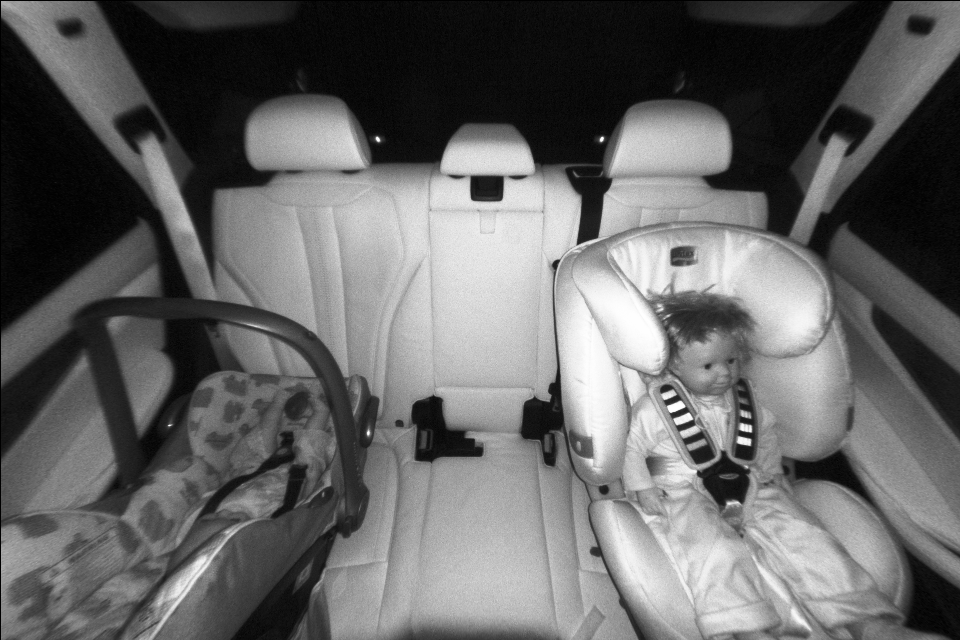
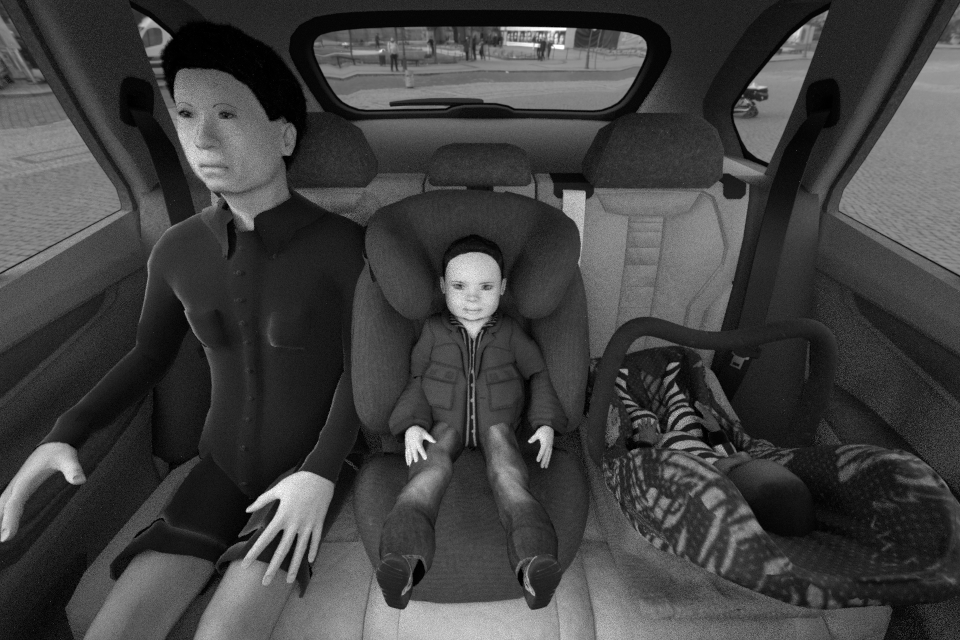
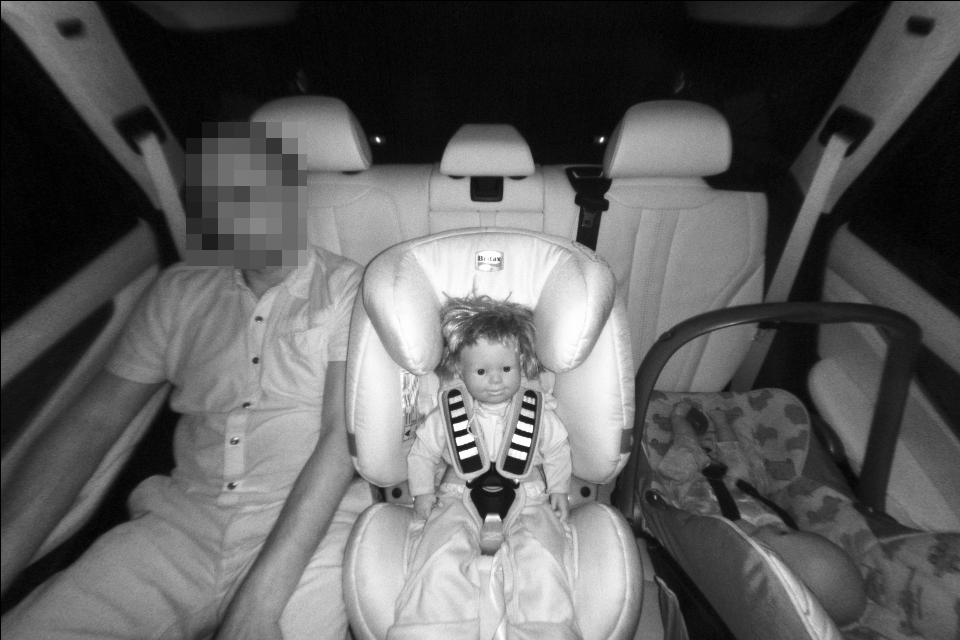
About Spotlight on Young Researchers
Spotlight on Young Researchers is an FNR initiative to highlight early career researchers across the world who have a connection to Luxembourg. The campaign is now in its 5th year, with 60+ researchers already featured. Discover more young researcher stories below.