BACK TO RESEARCH WITH IMPACT: FNR HIGHLIGHTS
A high number of people experience experiences depression in older age. Factors such as childhood conditions, adult life trajectories, and gender play a role, while key influential factors include childhood material deprivation, adult life stability, and education level. By combining machine learning with life course events, researchers have been able to determine predictors for depression, such as life instability and low utilisation of dental care, highlighting the complex interplay life events have on mental health.
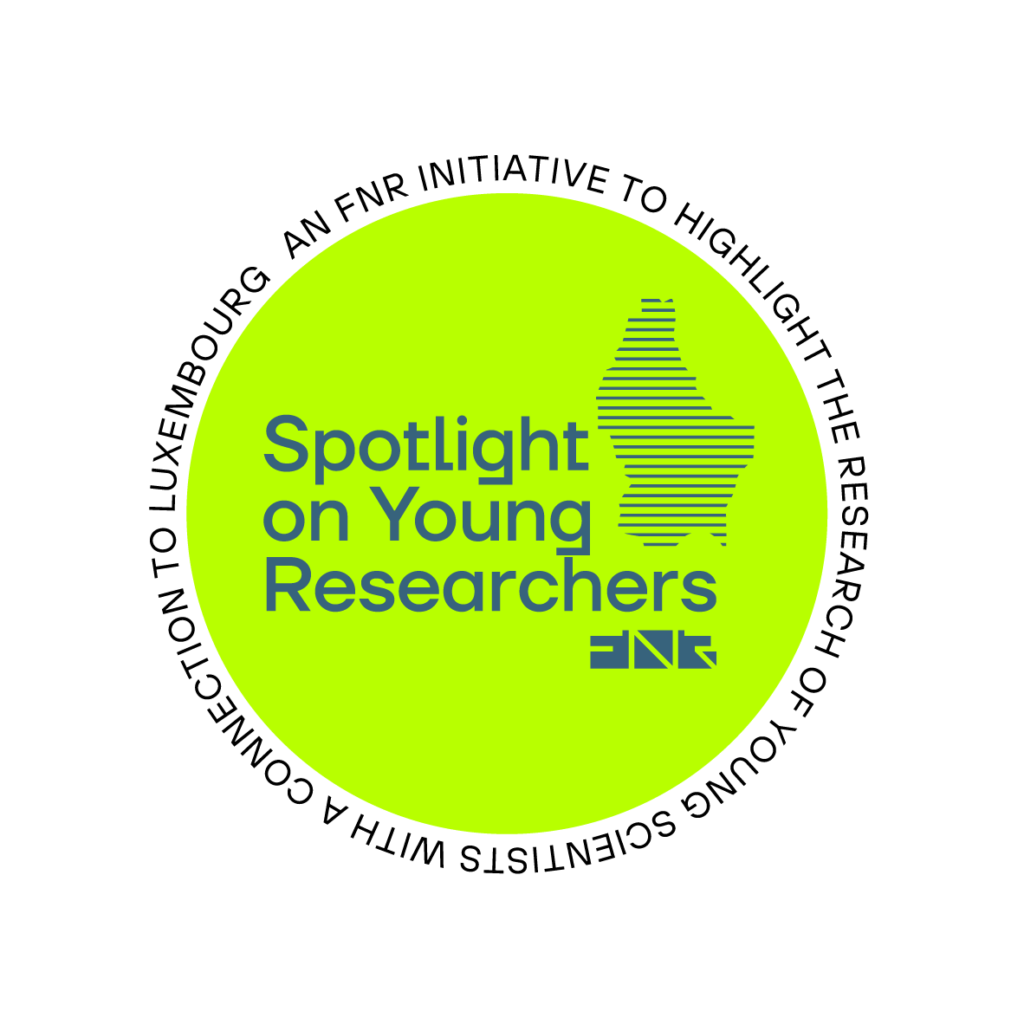
Machine learning helped improve the understanding and prediction of depression in older age – by combining it with life course data. Studies such as these emphasise the important role early-life conditions, stable adult life trajectories, and healthcare access play in predicting depression. Complementing traditional methods, this approach provides new insights into the long-term effects of life events and conditions on mental health.
But: The data relating to individual life course is vast and identifying key life events and conditions that significantly influence depression risk is complex a matter, because of the interconnectedness of genetic, environmental, and personal factors.
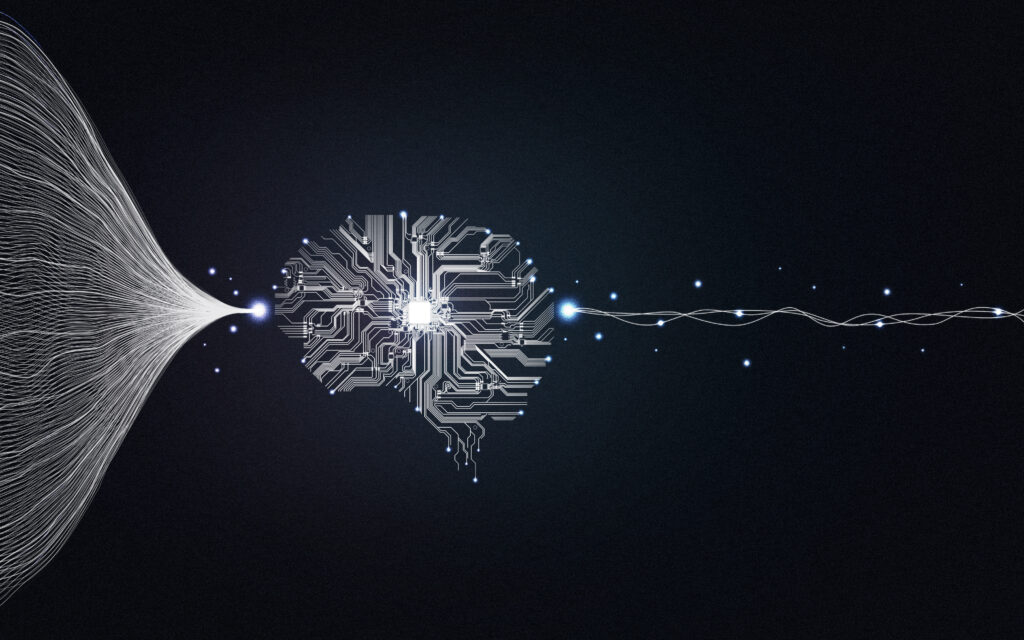
“Limitations in data accuracy, recall bias, and the complexity of life course information pose significant challenges. Additionally, the diversity in healthcare systems and social welfare interventions across different regions complicates the generalizability and effectiveness of predictive models. Addressing these data and methodological challenges is crucial for advancing the field. ”Carlotta Montorsi social scientist and economist who takes a computer science approach to her research.
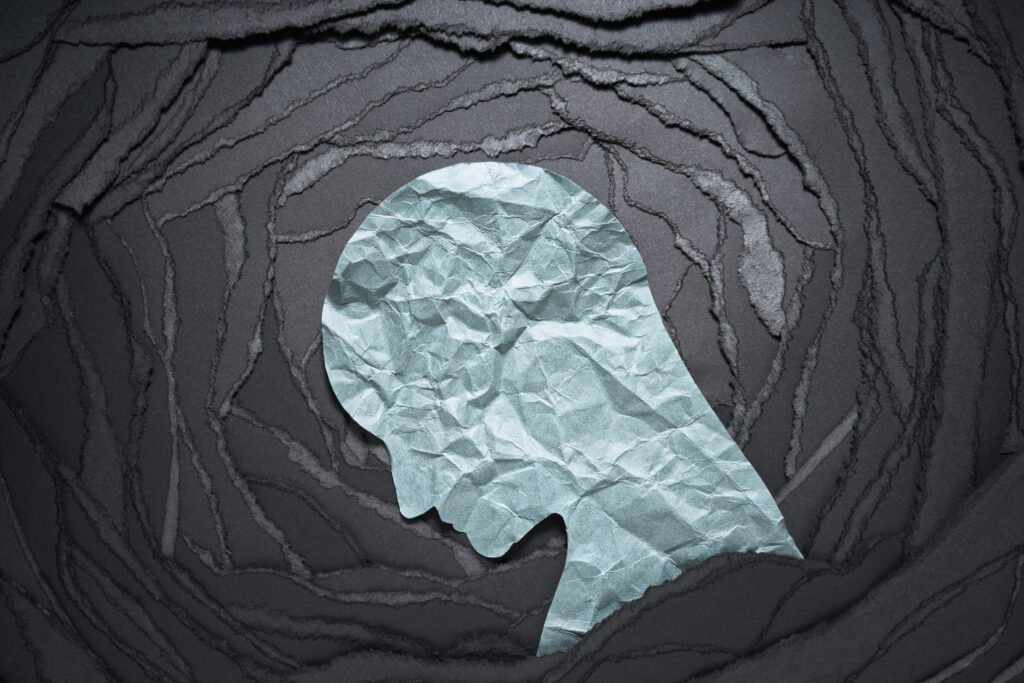
Carlotta’s research makes use of state-of-the-art machine learning algorithms on life course data to predict depression in old age. Integrating detailed life trajectories and health data, she is able to gain insight into how early-life conditions, adult life experiences, and healthcare access contribute to depression risk – an approach providing a nuanced understanding of depression’s multifactorial nature, while also identifying potential intervention points.
“We have identified key predictors of old age depression, including life instability and dental care access, highlighting the importance of comprehensive healthcare and stable life courses in mental health prevention. We also highlight that depression in female is more foreseeable than for male. ”Carlotta Montorsi social scientist and economist who takes a computer science approach to her research.
Carlotta Montorsi is a Social Scientist and Economist by training, but dedicated a large part of her PhD to learning and applying methodologies from Data and Computer Science. She successfully defended her PhD, which took place at the Luxembourg Institute of Socio-Economic Research (LISER), in November 2024.
MORE ABOUT CARLOTTA MONTORSI
Describing her research in one sentence
“My project leverages machine learning and detailed life histories to predict and understand the complex causes of depression in the elderly, aiming to improve mental health interventions.”
On her research, peer to peer
“Utilizing supervised machine learning models, the research analyses data from the Survey of Health, Ageing, and Retirement in Europe, comparing six algorithms against different life course data configurations. It demonstrates that semi-structured life sequences yield the most accurate depression predictions. This innovative approach not only offers predictive insights but also enhances our understanding of the longitudinal impact of life events on mental health outcomes.”
What fascinates her about research
“I am fascinated with the interplay between socio-economic factors and individual well-being; I chose to delve into Economics and Computational Social Sciences at LISER and the University of Luxembourg. My passion lies in bridging big data and machine learning to address complex issues like discrimination, mental health and the ageing of the population.”
“I am captivated by the potential of machine learning and big data analytics to unveil patterns within societal and economic data, offering insights that drive impactful policies and improve individual lives.”
Mentors with an impact
“During my PhD, I was fortunate to have Alessio Fusco,Chiara Gigliarano, Philippe Van Kerm, and Stephane Bordasas as my advisors. Alessio kept my research objectives in focus and provided invaluable support, while Chiara’s encouragement and trust guided me through moments of uncertainty early in my career. Phiippe’s insightful feedback, to-the-point questions, and “holistic” vision have been fundamental in better framing my research questions. Stéphane’s sparkling and positive personality, paired with the opportunities to interact with the Computer Science department, has been highly beneficial for developing machine learning skills. Their combined mentorship has been instrumental in shaping my development as a researcher and has laid a solid foundation for my future endeavors.”
Where she sees herself in 5 years
“In five years, I see myself leading innovative research projects that bridge computational techniques with research questions from economics and social science, potentially within an academic or research oriented institution, to contribute further to societal well-being and economic understanding.”
Related Funding Instruments
Related highlights
Spotlight on Young Researchers: Advancing ecosystem monitoring with remote sensing and innovative models
The health of terrestrial ecosystems is intricately linked to the sustainability and stability of society: forests, grasslands, and cropland, play…
Read more
Spotlight on Young Researchers: Unravelling the role of calcium signalling to overcome melanoma drug resistance
Cutaneous melanoma is the most serious type of skin cancer and the sixth most frequent cancer in Europe. Despite progress…
Read more
Spotlight on Young Researchers: Advancing sustainable land use
Industry, agriculture and waste disposal all cause soil pollution, a threat to both ecosystems and human health. Environmental scientists and…
Read more
Spotlight on Young Researchers: Toward greener AI
Deep learning has seen an explosive growth and as it is now being relied upon in areas such as healthcare,…
Read more
Spotlight on Young Researchers: Probiotics to the rescue
Billions of living microorganisms live in the human gut microbiome. Research has shown that an imbalanced microbiome plays a role…
Read more
Spotlight on Young Researchers: How multilingualism impacts learning numbers and mathematics
In an increasingly multicultural and multilingual society – especially in Luxembourg – it is key to ensure an educational system…
Read more