BACK TO RESEARCH WITH IMPACT: FNR HIGHLIGHTS
BACK TO RESEARCH WITH IMPACT: FNR HIGHLIGHTS
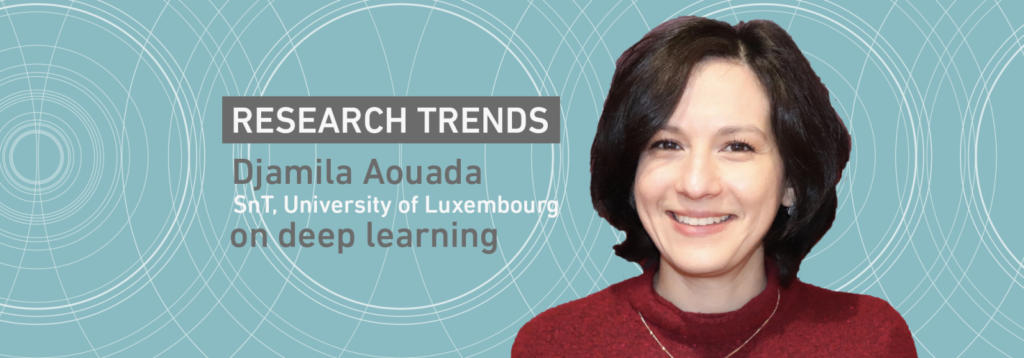
As part of a new series, the FNR speaks to five experts about research trends in their domain. Deep learning has allowed scientists to make computers function in a way much closer to how humans think than ever before: Djamila Aouada from the SnT at the University of Luxembourg explains how deep learning works, and her efforts to make it 3D capable.
Do you still unlock your mobile phone with a PIN? Or do you already use your fingerprint? Both of these identification methods may soon be outdated.
“Machines will very soon be capable of identifying people from the shape of their face and body,” says Dr Djamila Aouada
Aouada adds: “Machines will recognise whether a facial expression really is that of the phone owner, or whether a motion sequence truly belongs to the person who is requesting entry into a secure area. Maybe in the not too distant future we won’t even need passports anymore because the airport security systems will be able to match posture, gait and facial movements to a specific person.”
This revolution is becoming possible thanks to a certain technology that has been making computers smarter for several years now, called deep learning. There is still a multitude of technical, legal and ethical issues to be resolved before it is ready to be used.
Yet, Aouada, who heads the Computer Vision Team in the SIGCOM group at the Interdisciplinary Centre for Security, Reliability and Trust (SnT) of the University of Luxembourg, is eagerly working on it with her team.
With deep learning, scientists have managed to make computers function in a way that is much closer to how humans think than was ever the case before. “Deep learning runs on different levels of so-called perceptrons,” Aouada explains: “These are nodes in multilayer networks. The degree of abstraction of the categories processed becomes greater from one network level or layer to the next.”
The higher the network level, the more abstract the features
What exactly does that mean? On a relatively low level, the computer processes simple isolated shapes such as the top contour of an upper lip or the arc of an eyebrow. The higher up in the network levels, the more abstract and complex the extracted features become.
At these levels, it is about more than just lines or dots. Moreover, the network learns the features describing a “face” category from which it can then recognise any face, just like a person.
Deep learning’s immense power, Aouada states, lies in the fact that it runs automatically – provided it has enough data to work with.
In the case of two-dimensional face recognition, there is certainly plenty of data, given that millions – if not billions – of photos of faces exist on the social networks such as Facebook. But that is not much help for the technical application of personal identification, as the researcher explains:
“If a computer recognises two-dimensional faces, then you can simply hold a picture up to the camera. Even a PIN is more secure than that. Face recognition therefore has to be three dimensional, and even more has to include the facial movements.”
Making deep learning truly 3D-capable
That is easier said than done. Not only because not enough three dimensional data exist yet, but also because deep learning is still not even technically capable of efficiently processing three-dimensional data.
Currently, Aouada’s team works around this by transforming data down from three to two dimensions. “But that can’t be the solution, long term,” the computer scientist argues: “Which is why we are working on making deep learning truly 3D-capable.”
Intense cooperation with jurists and social scientists is necessary
Only very few research groups worldwide have begun to tackle this subject so far; it is a very young research field. Nevertheless, Aouada is convinced that “in four to five years, a mature solution will be available.” Then, theoretically, there would be nothing standing in the way of checking in at the airport without a passport.
Of course, what is technically feasible has not all been clarified legally or accepted socially. Aouada declares:
“We must cooperate increasingly with jurists and social scientists in order to resolve the pertinent issues. The SnT Centre and the University of Luxembourg, with its interdisciplinarity, is the ideal place for that.”
About Djamila Aouada
Djamila Aouada is Research Scientist at the Interdisciplinary Centre for Security, Reliability, and Trust (SnT), at the University of Luxembourg, and head of the Computer Vision lab in the SIGCOM group. She received the State Engineering degree (Ingéniorat d’État) in electronics in June 2005, from the École Nationale Polytechnique (ENP), Algiers, Algeria, and the Ph.D. degree in electrical engineering in May 2009, from North Carolina State University (NCSU), Raleigh, NC, USA. Dr. Aouada has been leading the computer vision activities at the SnT since November 2009. She attracted several national, industrial and European projects. She has co-supervised 5 PhD theses and is currently the main supervisor of 6 PhD candidates. Dr. Aouada has worked as a consultant for multiple renowned laboratories (Los Alamos National Laboratory, Alcatel Lucent Bell Labs., and Mitsubishi Electric Research Labs.). Her research interests span the areas of signal and image processing, computer vision, pattern recognition and data modelling. Dr. Aouada is member of the IEEE Signal Processing Society, INSTICC, and the Eta Kappa Nu honor society (HKN). She has served as the Chair of the IEEE Benelux Women in Engineering Affinity Group from 2014 to 2016. She regularly serves as a reviewer for journals and conferences, including CVPR, IEEE TIP, CVIU, and SigPro. She is the coauthor of four IEEE best paper awards.
OTHER HIGHLIGHTS FEATURING DJAMILA AOUADA
OTHER ARTICLES IN THIS SERIES
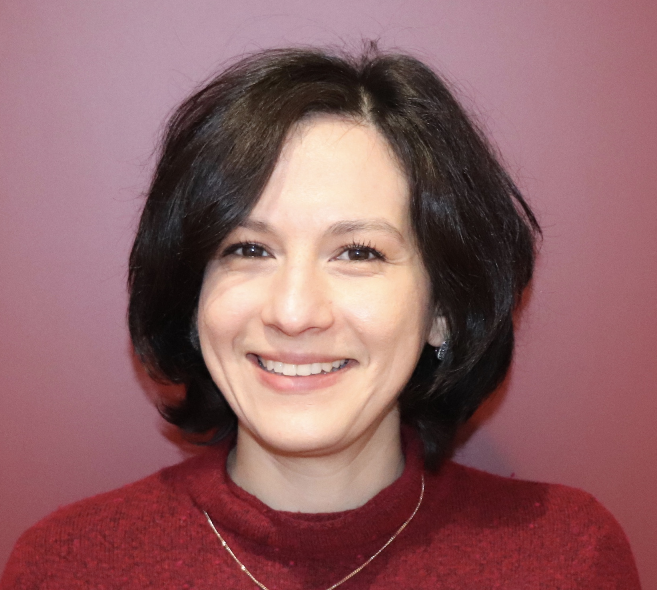
In hot demand: expertise in deep learning
The concept of deep learning has already existed for quite some time. However, it has only become technically feasible since the positive explosion in computing power in recent years. This has enabled us to process gigantic sets of data.
Now, our most scarce resource is the expertise to drive this research forward. Strong competition for undergraduates and postgraduates has broken out among research groups at universities, and even R&D departments of companies. Deep learning is such a young development that it is not even being taught yet, at least not to any great depth, at the universities.
Dr Aouada is acutely aware of that: “We welcome anyone who, alongside a classical training in engineering and in the computer sciences, can already demonstrate experience in deep learning.”